About BroaderBiz Inc.
BroaderBiz Inc. provides solutions to promote the spread of AI that is useful to society, with its role as an AI enabler and integrator that promotes AI utilization at the core of its business. Founded in July 2017 with the aim of launching businesses that implement and utilize image analysis technology, BroaderBiz’s core members have over 20 years of experience providing cutting-edge web and image processing technology solutions to major companies in Japan and around the world. Going beyond just spreading AI technology as basic research, BroaderBiz wants to create AI that contributes to the lives of working people by applying it to real operations as a system integrator.
Monitoring Previously Difficult-to-Track Chronological “Human” Behavior to Protect Food Safety
A major problem in the food products industry in recent years is food contamination, including foreign bodies in food and the malicious addition of drugs and other substances. Another important theme is worker safety. In the past, these sorts of incidents were prevented and work safety ensured by managers keeping an eye on workers, with countermeasures taken based on the status of work progress and any problems that occur. More recently, video recordings of work operations are also used.
However, these sorts of traditional approaches have problems, such as:
- It is not easy for managers to continuously monitor long shifts of factory work
- Since workers wear uniforms, hats, and masks, it is difficult to visually identify individuals
- Inability to quantitatively monitor and record work data makes it difficult to compare with the past
- Video recordings may be used as evidence after the problem has occurred, but it is unsuitable for immediate response
What BroaderBiz began focusing on was coming up with ways to prevent problems from getting worse by using AI-powered systems to “watch over” manual labor by humans, compare against quantified statistics, and immediately identify abnormalities that deviate from standard behavior.
According to BroaderBiz CCO Makoto Aoki, “We thought it was important to quantify workers’ behavior, which was traditionally visually monitored and managed by humans, and create a quantitative base for work management. ” Since these initiatives focus on major themes like the mislabeling of food and workstyle reforms, they were recognized as NEDO Joint Development Support Projects.
Chose Elastic for its End-to-End Capabilities from Data Collection to Analysis, Visualization, and Notification
In August 2018, BroaderBiz began studying what technology and specific products were required to build this “Work Environment Monitoring System Powered by AI Image Analysis.” One key task in this was to develop machine learning functionality capable of deriving patterns of normal work behavior based on chronological data. Having already been an Elastic user, including Elasticsearch and Kibana, BroaderBiz began assessing Elastic products in comparison with other companies’ BI tools. In the end, they discovered that Elastic was superior in many respects.
“We were already familiar with Elasticsearch, and we used Kibana for its dashboard functionality. Since machine learning functionality was being offered, we considered using Elastic compared to other possible candidates from other companies. Some of Elastic’s biggest advantages were the ease of linking up with other devices and systems, the highly efficient development that this helps enable, and the end-to-end features ranging from data collection to aggregation, analysis, visualization, and notification (linkage),” said Aoki.
In this case, the systems involved include IP surveillance cameras equipped with AI boards that film the workplace, edge devices linked up with these cameras, edge data management systems including video and image storage, and cloud systems for further data management and visualization. High connectivity and open architecture are essential to bringing together all of these devices and systems, and the Elastic Stack satisfied all of these requirements. Of course, compared to using these products with their individual features in isolation, combining them together into normal development or integration processes can make development dramatically more efficient. Moreover, using Elasticsearch, Kibana, Machine Learning, and Alerting together enables comprehensive, end-to-end functionality. BroaderBiz thus made the decision to use Elastic Stack in October 2018, launched projects to implement the technology, and finally went live with the new system incorporating Elastic Stack in February 2019.
Using Machine Learning to Model Chronological Data Based on Behavioral Patterns Over Time
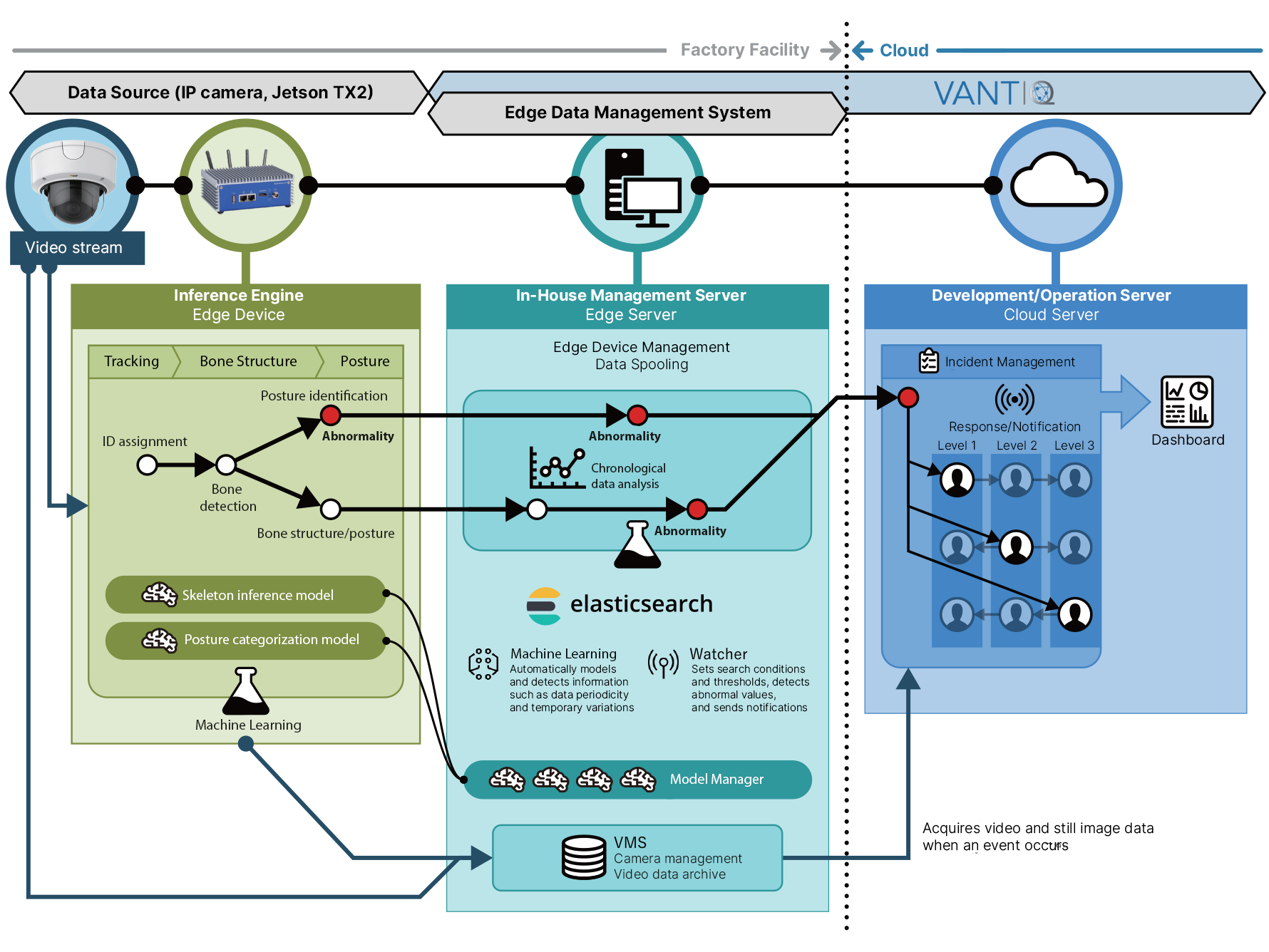
The “Work Environment Monitoring System Powered by AI Image Analysis” assigns unique IDs to individual workers as they move around the screen, captured by AI board-equipped surveillance cameras set up in factories and GPU-equipped edge devices. Next, the system identifies each person’s nose, neck, and waist to estimate skeletal structure. By matching skeletal structure coordinates with posture patterns, the system determines the posture of each worker. If the system then detects a remarkably unnatural posture that continues for several seconds, it will raise a flag and send out an incident alert. Incident data is sent in JSON format using the MQTT protocol, through administrative edge servers set up in factories to a cloud system for data management and visualization purposes.
In addition to this one system process that identifies postures on a momentary basis, there is another system process that looks at work over time. In this case, the system looks at repetitive worker movements in the course of their ordinary work in terms of skeletal and posture data, aggregates that data by time and work pattern, and generates a model using Elastic’s Machine Learning functionality. Around one month after the data begins to be input into the system, a model will be automatically generated. Thereafter, the system will compare against this model to determine abnormalities that deviate from normal movements. For example, if the system detects non-regulation procedures, contamination by foreign objects, or unauthorized entry to the worksite, it will be able to send out an incident alert.
Easy to Implement and Use. Detection of Abnormalities Using Behavioral Analysis Based on Chronological Data
Regarding the benefits of implementing Elastic Stack’s Machine Learning, Aoki said: “Typically, connecting and integrating various separate features requires a lot of work. For example, when connecting a database with a machine learning service, it is usually necessary to check on, consider, and otherwise deal with I/O for each. However, the Elastic Stack is one integrated package of features that eliminates the need for any of that work or consideration that are typically necessary. Additionally, being able to use machine learning to automatically model workflows over time had a huge impact in terms of the amount of manual labor saved,” he emphasized. He also said that they were able to achieve the core functions of this system “in line with initial expectations,” including behavioral analysis and detection of abnormalities using chronological data and the sending of incident alerts.
Elastic Stack provides features in a single package, eliminating the need for any consideration of connectivity between features. Additionally, machine learning enables automatic model generation without human involvement. In this way, it was hugely beneficial to be able to lower the burden of implementation and administration while achieving behavioral analysis and abnormality detection using chronological data in line with expectations.
Regarding the advantages for companies that introduce this system, Aoki emphasized that “Using AI to quantify worker behavior, and storing that data so it can be reviewed later to understand behavior and operational status, not only makes it easy to detect abnormal behavior and look into the causes of problems when they occur, it also helps the implementing company prove that there are no problems in company operations.”
Deploying the System Elsewhere While Maximizing Use of the Elastic Stack
BroaderBiz heavily benefitted from Elastic Stack features including Machine Learning in developing this system, and the company is actively considering using Elastic Stack in its plans to deploy this system elsewhere in the future. “In this case, we developed the system for the factory of a food products company, but it can be implemented for any type of job in which workers’ tasks are standardized. For example, we think it could also be applied to ‘watch over’ work done in the kitchen of a franchised restaurant,” said Aoki.
With BroaderBiz’s approach to system development, it is possible to monitor the situation at the workplace based on video alone, without complicated sensor installation or other tasks that involve a lot of work. Moreover, in some cases it may be possible to use video footage from existing cameras, which would make it possible to implement this system with even lower initial costs. This means there is a wide range of potential use cases.
In closing, Aoki emphasized the following: “Watching over manual labor in this way requires both instantaneous judgment and judgment based on observation over time. In this case, we were able to use Elastic Stack to achieve a solution focusing primarily on observation over time, and I feel this will prove to be an extremely important step forward in BroaderBiz’s business in the future.”
BroaderBiz continues to utilize AI to provide solutions to various problems facing customers. Elastic Stack is one important element underlying those initiatives, and it will likely continue be used to its fullest in the future ahead.