How ML-driven search is helping ecommerce sites boost customer loyalty
Search abandonment costs $333 billion a year, but retailers are (machine) learning how to keep customers happy
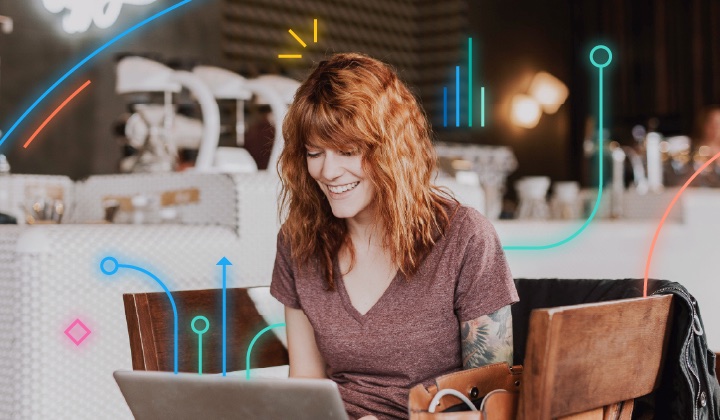
It's a great time to be an online retailer. But it's also more challenging than ever.
In 2022, global ecommerce sales are projected to exceed $5.4 trillion, continuing a pattern of explosive growth that was accelerated by the pandemic. Yet, competition is intense and expectations are high, while customer loyalty is extremely fluid. If people can't easily find what they're looking for on your site, they'll buy it somewhere else — and they might never return.
A Harris Poll survey of 10,000 customers worldwide found that 76% abandoned a retailer after failing to find a product they wanted. Roughly half ended up buying the product elsewhere. Per the study, search abandonment costs U.S. retailers an estimated $333 billion each year.
It's why forward-thinking e-tailers are turning to machine-learning (ML) technologies to provide more relevant and personalized ecommerce search platform results.
Gauging shopper intent
More than ever, ecommerce search needs the ability to understand customer intent. Research by the Baymard Institute reveals that 70% of the top 149 ecommerce sites in the U.S. and Europe are unable to return relevant results for product synonyms (for example, "notebook" or "portable pc" instead of "laptop"). More than a third fail to produce relevant results if shoppers misspell just a single character in a product title.
Ecommerce sites need to understand what customers are really looking for. While traditional synonym dictionaries can produce functional results for searches employing alternate descriptors, and analytics can suggest additional items related to those shoppers are searching for, AI-based semantic search is needed to make the next big leap in producing more relevant results, which in turn leads to a more positive customer experience.
Semantic search comprises several complementary technologies, including machine learning, natural language processing (NLP), and vector search.
Natural language processing captures the meaning and context of language, allowing search engines to understand search queries written in everyday vernacular (such as “what tools can I use to fix a leaky faucet?”). It works by turning text into numbers (aka vectors). Vector search can then identify words or images that are semantically similar (for example, film, cinema, movie) by finding vectors that are numerically similar.
But identifying relevant products within databases containing millions of entries can be extremely complex and time consuming. A vector search technique known as approximate nearest neighbor (ANN) can speed searches dramatically by pre-indexing data entries into groups of related concepts.
All together, these technologies allow ecommerce search engines to determine context and customer intent.
For example, a semantic search will identify instances of notebook computers and portable PCs as close matches when querying for laptops. Additional NLP techniques can take the interaction to the next level, from very specific queries to empowering the customer to describe what they want; even if they don’t know the name of the product, NLP-powered search will return a set of likely results. And machine learning models can classify additional attributes (like customer sentiment) that you can use to personalize your search results.
Watch our webinar on 2022 Enterprise Search Trends: ML-powered search relevance
Predicting and personalizing
Once you understand customer intent, you can personalize the shopping experience for each shopper and even predict future buying behavior.
Machine learning can take multiple contextual factors into account — not merely the products being searched, but also the customer's geographic location, time of year, purchase history, affinities with similar customers, and so on — to make recommendations for additional purchases. Again, this process works by turning each of these factors into their own vectors, and using vector search to identify similarities between them.
Improving site search allows for better use of predictive analytics and vice versa. If you know that someone is searching for, say, bathing suits, they may also be more inclined to purchase beach towels, based on the behavior of other shoppers who made similar transactions.
Studies by ecommerce platform vendor Barilliance show that personalized product recommendations account for nearly a third of all ecommerce revenue, and can increase average order value by more than 3X. Shoppers who engage with a product suggestion are 70% more likely to convert, per studies by Kibo.
Conversely, bad recommendations can cost an etailer greatly. Surveys by shopper engagement platform Bazaarvoice show that nearly four out of ten U.S. shoppers will cease doing business with retailers whose product suggestions don't make sense to them.
Greater insights, less friction
Intelligent search, combined with analytics, can also be a great source of insights for improving the customer experience. Used together, they can identify items your customers are looking for that your site doesn't yet carry, or flag potential supply chain shortfalls based on higher demand for related products.
Frictionless commerce and interactions with more personalization are the key to online success. Allowing customers to search using natural language that's typo tolerant and synonym friendly is a great way to deliver more relevant results, streamlining the shopping experience. NLP and machine learning can also help you analyze customer sentiment, showcase positive reviews and other helpful information, answer questions, and recommend related products, boosting overall revenues.
Ultimately, deploying more intelligent search can make the difference between converting a new customer or losing a loyal one.
Read this next: Product over Price: The critical role personalization plays in converting online searches to sales