The role of generative AI in higher ed IT
Find the answers you need by securely integrating your university’s data with public LLMs and Elasticsearch
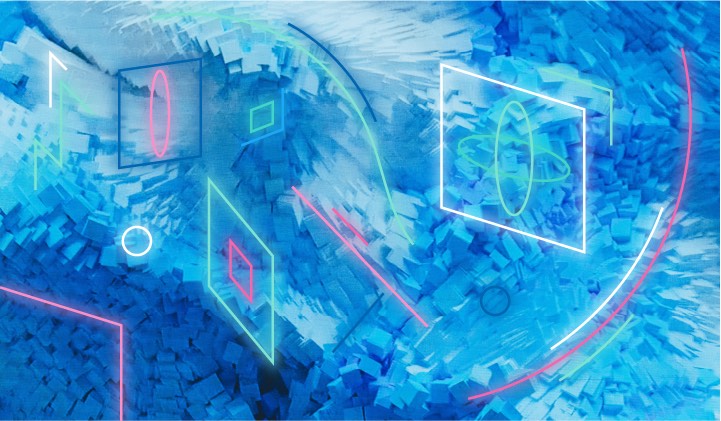
According to an EDUCAUSE survey from April 2023, 83% of respondents say that generative AI will profoundly change higher education in the next three to five years.
The academic community has been quick to question and imagine the impacts of generative AI on teaching and learning. Scholars and decision makers are exploring what generative AI means for personalized learning, integrity and ethics, and academic research, as well as preparing students for an AI-powered workplace.
In addition to pedagogical impacts, generative AI also has the potential to transform the IT landscape at universities. Specifically, when generative AI applications are used with a university’s internal data, they can produce hyper-relevant, context rich outputs that can increase productivity and job satisfaction and lead to better student outcomes using generative AI search engines. Let’s explore how universities can use generative AI for the benefit of their students and staff, and IT infrastructure.
Powering university IT operations with generative AI + private data
The key to harnessing the power of generative AI for university IT operations is to securely integrate it with your own data. Students and educators may be familiar with using publicly available generative AI applications such as ChatGPT, which are built and trained on publicly available data, in their teaching and learning. But to see operational and personalized results, using this private data is essential.
When generative AI forms outputs based on your own data instead of publicly available data, it can be much more accurate and personalized. A platform such as Elasticsearch® can serve as a secure bridge between the large language models (LLMs) of your choice and your private data. Once this link is established, many use cases can be enabled based on your goals and priorities.
Together, Elasticsearch + generative AI + your private data can bring context to your data, helping you find the answers you need, quickly. This manifests itself in several areas across a university, including:
- Boosting productivity and employee satisfaction. Think how much time university employees spend simply looking for information, whether it’s documents, student data, resource utilization, class schedules, or more holistic data-driven insights and patterns. This is essential information that helps them do their jobs, but it probably isn’t how they want to spend their time. When you ingest your data into Elasticsearch and connect it to a generative application, you can significantly reduce the time previously spent manually searching through the depths of your intranet and shared drives. Generative AI can also help automate IT workflows and create documentation on processes and events.
- Personalizing digital support services. In addition to internal staff, universities have large volumes of students, educators, and prospective students and parents browsing your externally facing digital properties for information on the application process, course catalog, enrollment instructions, financial aid, sports schedules, and more. Enabling generative AI capabilities with your university’s data can surface detailed information tailored to specific audiences or requests, increasing the likelihood that stakeholders find what they need quickly (and reducing the work of your support teams).
- Strategically tracking and allocating resources. Using your data and generative AI, you can detect and visualize data patterns across your university, tracking things like how physical spaces are being used, IoT device resource utilization, virtual learning connections, and more. This aggregated data can enable the right people to make the right decisions in real time, ultimately leading to more strategic and informed resource allocation.
- Streamlining cybersecurity. Universities can put generative AI to work for cybersecurity purposes as well. Security teams can enable the Elastic® AI Assistant for personalized help on tasks like alert investigation, incident response, and query generation. The Elastic AI Assistant offers users prebuilt, recommended prompts as well as specific context for LLMs.
Get started with generative AI by strengthening your IT foundation
With generative AI taking the world by storm, it’s easy to get swept up in the possibilities and harder to land on concrete steps to turn possibility into action. As you look to implement generative AI in your university’s tech stack, a good place to start is by doing some legwork in prepping your data.
McKinsey, a global technology strategy company, recently published a new generative AI guide, which offers some practical insight for leaders interested in getting started with generative AI. McKinsey recommends that CIOs, CDOs, and CTOs should together focus on three steps — all of which can be accomplished with the Elasticsearch platform.
1. Prep your data for generative AI
As with many aspects of IT modernization and process augmentation, big changes start with data. The McKinsey team suggests that before implementing generative AI into their tech environment, tech leaders should “categorize and organize data so it can be used by generative AI models. Tech leaders will need to develop a comprehensive data architecture that encompasses both structured and unstructured data sources. This requires putting in place standards and guidelines to optimize data for generative AI use.”
Elasticsearch is a unified data platform that can ingest all data types and correlate them for integrated queries, analysis, and visualization. Once your data is in the platform, you can use APIs to connect it to your LLM of choice to reap the benefits of generative AI on top of your private data.
2. Ensure system scalability
Additionally, McKinsey advises technology leaders to ensure their systems can scale to handle increasing data volumes that inevitably come with generative AI implementation.
This is another area where having a powerful platform like Elasticsearch can provide a solid foundation. Elastic regularly works with organizations that handle petabytes of data — powering the US government’s Continuous Monitoring and Diagnostics (CDM) dashboard that collects data from hundreds of federal agencies, as well as some of the world’s largest supercomputers.
3. Consider vector search
McKinsey’s third priority for tech leaders ramping up for generative AI is to “prioritize the development of data pipelines to connect generative AI models to relevant data sources that provide ‘contextual understanding.’” One of these approaches is using vector databases.
Again, the Elasticsearch platform is intrinsically suited to help here, given vector search technology. Elastic’s vector search leverages machine learning (ML) to capture the meaning and context of unstructured data, such as text and images, and then transforms it into a numeric representation. Vector search enables users to search by what they mean — without having knowing an exact keyword — delivering answers based on similarity search. This flexibility increases the accuracy and relevancy when applied to generative AI applications.
Learn more about Elastic’s approach to generative AI:
The release and timing of any features or functionality described in this post remain at Elastic's sole discretion. Any features or functionality not currently available may not be delivered on time or at all.
In this blog post, we may have used or referred to third party generative AI tools, which are owned and operated by their respective owners. Elastic does not have any control over the third party tools and we have no responsibility or liability for their content, operation or use, nor for any loss or damage that may arise from your use of such tools. Please exercise caution when using AI tools with personal, sensitive or confidential information. Any data you submit may be used for AI training or other purposes. There is no guarantee that information you provide will be kept secure or confidential. You should familiarize yourself with the privacy practices and terms of use of any generative AI tools prior to use.
Elastic, Elasticsearch, ESRE, Elasticsearch Relevance Engine and associated marks are trademarks, logos or registered trademarks of Elasticsearch N.V. in the United States and other countries. All other company and product names are trademarks, logos or registered trademarks of their respective owners.