Elasticsearch: Build an AI-powered search experience
Overview
Introduction to Elasticsearch
Get familiar with Elasticsearch with an overview on how to ingest, and view your data with Elastic Cloud.
As you ramp up on Elastic, you’ll use the Elasticsearch Relevance Engine™ (ESRE), designed to power AI search applications. With ESRE, you can take advantage of a suite of developer tools including Elastic’s textual search, vector database, and our proprietary transformer model for semantic search.
Elastic offers a variety of search techniques, starting with BM25, the industry standard for textual search. It provides precise matching for specific searches, matching exact keywords, and it improves with tuning.
As you get started on vector search, keep in mind there are two forms of vector search: “dense” (aka, kNN vector search) and “sparse” such as Elastic’s Learned Sparse Encoder (ELSER).
Elastic also offers an out-of-the-box the Learned Sparse Encoder model for semantic search. This model outperforms on a variety of datasets, such as financial data, weather records, question-answer pairs, among others. The model is built to provide great relevance across domains, without the need for additional fine tuning.
Check out this interactive demo to see how search results are more relevant when you test Elastic's Learned Sparse Encoder model against Elastic’s textual BM25 algorithm.
In addition, Elastic also supports dense vectors to implement similarity search on unstructured data beyond text, such as videos, images, and audio.
The advantage of semantic search and vector search is that these technologies allow customers to use intuitive language in their search queries. For example, if you wanted to search for workplace guidelines on a second income, you could search for "side hustle", which is not a term you're likely to see in a formal HR document.
In this guide, we'll demonstrate how to create an Elastic Cloud account, ingest data with the Elastic web crawler, and implement semantic search in just a few clicks.
Let's get started
Create an Elastic Cloud deployment
Get started with a 14-day trial. Once you go to cloud.elastic.co and create an account, follow the steps below to learn how to launch your first Elastic stack in any one of our 50+ supported regions globally.
If you click on Edit settings you can choose a cloud provider, including AWS, Microsoft Azure, or Google Cloud. Once you select your cloud provider you'll be able to select the relevant region. Next, you have the option to choose between a few different hardware profiles so you can better customize the deployment to suit your needs. Plus, the latest version of Elastic has already been preselected for you.
While your deployment is being created, you'll be given a username and password. Be sure to copy or download this as you'll need it when you install your integrations.
Configure the Elastic web crawler
Now that you've created your deployment it's time to get data into Elastic. Let's do this by using Elastic's web crawler. First, under the Search tab, you'll select the tile, Build an AI-powered search experience.
Next, to set up semantic search, you'll see a page where you can get started with either of the following:
- Elastic Learned Sparse Encoder
- Vector Search
- NLP Enrichment
All of these capabilities and more are part of the Elasticsearch Relevance Engine (ESRE).
For the purpose of this guide, let's go through setting up semantic search with both, the Elastic Learned Sparse Encoder and Vector Search.
NOTE: If you're getting started with semantic search and want to search text, you should try the Elastic Learned Sparse Encoder guide first. The kNN Vector Search guide may be more suitable for users who meet some of these criteria:
- Have access to a data science or data science skill set
- Have determined the built-in Elastic Learned Sparse Encoder semantic search model will not cover their use-cases
- Are experienced in comparing embedding models, and potentially fine-tuning ML models
- Are aware that fast kNN search may require significant RAM resources
If you're ready to get started, select your preferred method to build an AI search-powered application.
For both methods, you'll get started by selecting Create an index. From here you can select the web crawler to get started with ingesting your data.
To set up the web crawler, check out this guided tour or follow the instructions below:
Now create an index. For the purpose of this guide, we're ingesting blogs across elastic.co.
Once you give your index a name, select Create index. Next, you'll Validate Domain and then select Add domain.
After you add the domain in the lower right you'll select Edit so you can add a subdomain if needed.
Next, you'll select Crawl rules and add your crawl rules as seen below.*
Next, when you select your field later, some fields exceed the 512 token count, such as body_content. You should leverage Extraction rules to filter out only the relevant parts of the blogs.
When you select Extraction rules click Add content extraction rule.
Next, under Rule description give it a name that will help others understand what data this rule will extract. For the purpose of this guide, let's call it "main."
Now, select Apply to all URLs then choose Add content fields, and a flyout will appear. Fill out and select the following criteria below when the flyout appears.
- Document field:
- Field name: main
- Source:
- Extract content from: HTML element
- CSS selector or XPath expression: main
- Content
- Use content from: Extracted Value
- Store extracted content as: A string
Once you fill in this criteria click Save then Save rule.
Enrich your data using Elastic Learned Sparse Encoder
Get started with Elastic Learned Sparse Encoder, Elastic's out-of-the-box semantic search model, by following the instructions below.
To do this you'll select Pipelines and Unlock your custom pipelines by selecting Copy and customize at the top. Next, under Machine Learning Inference Pipelines, select Deploy to download the model and install it on your Elasticsearch deployment.
Once it deploys select Start single-threaded then + Add inference Pipeline. Next you'll do the following:
- Select new or existing pipeline
- Give it a name
- Finally, in the Select trained ML Model dropdown select ELSER Text Expansion then click Continue.
Now, you'll need to select the fields where you're going to apply the ELSER text expansion. Select "title" and "main" as source fields, then Add.
Next, click Continue.
Skip the Test your pipeline results step by clicking Continue, then Create pipeline.
Now that you've created your pipeline, select Crawl in the upper right corner then Crawl all domains on this index.
Working with Elasticsearch
Create a search query
Now it’s time to search for the information you’re looking for. There are two recommended ways to do this: and the first is by using Dev Tools. If you’re a developer who’s implementing search (i.e., for your web application) you should use the Dev Tools to test and refine search results from your indexed data.
Watch the below video for how to leverage Dev Tools.
The other way to create a search query is by leveraging the Search Application functionality as an endpoint for your application. If you want to create a search endpoint that you can send search requests from your own application where you can return search results, you should use the Search Application functionality.
Check out this short video below for a short walk through on how to use the Search Application functionality. You can also learn how by exploring this guided tour.
Advanced: Ingest and search using kNN vector search
When leveraging Elastic, you can take advantage of kNN vector search across your environment. As stated above, kNN vector search allows you to search across data other than text, such as images or audio.
After you have ingested data with the web crawler, next you'll need to load an embedding model into Elasticsearch to generate vectors for your data. Check out the video below to learn how.
To do this you'll select Pipelines and Unlock your custom pipelines by selecting Copy and customize at the top. Next, under Machine Learning Inference Pipelines, select Deploy to download the model and install it on your Elasticsearch deployment.
Once it deploys select Start single-threaded then + Add inference Pipeline. Next you'll do the following:
- Select new or existing pipeline
- Give it a name
- Finally, in the Select trained ML Model dropdown select Dense Vector Text Embedding then click Continue.
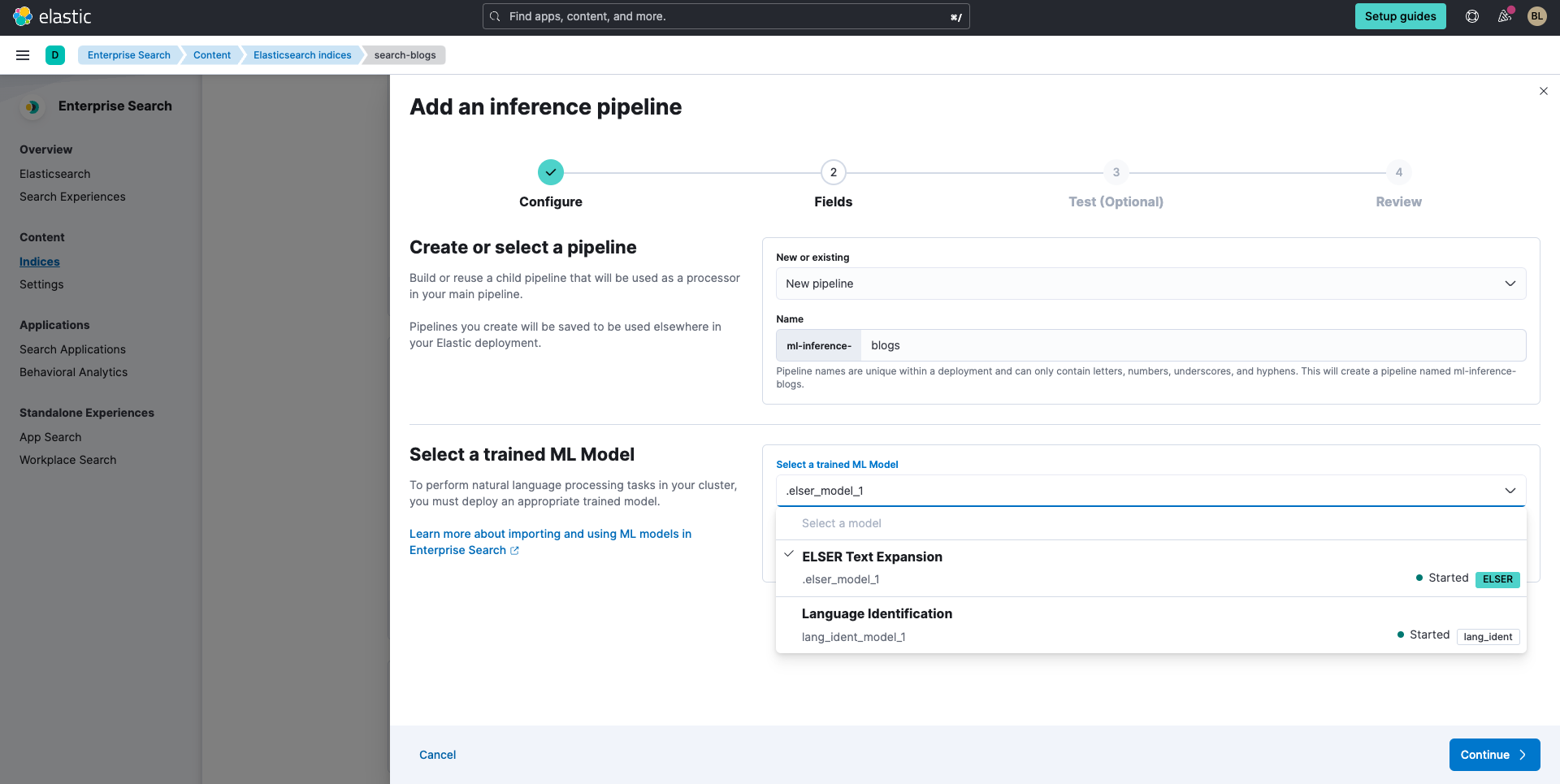
Now, you'll need to select the fields where you're going to apply the dense vector extension. Because you're using the web crawler, you'll have fields you can select by default. For the purpose of this guide, let's select title to start for a new field then select Continue.
Next select Continue, then Create pipeline.
Now you'll have to update the mapping for dense_vector field. (Note: with Elasticsearch version 8.8+, this step should be automatic.)
- In the navigation menu, click on Dev Tools. You may have to click Dismiss on the flyout if this is your first time opening Dev Tools.
- In Dev Tools in the Console tab, update the mapping for our kNN vector target field with the following code. You simply paste it in the code box and click the little arrow to the right of line 1.
POST search-blogs/_mapping
{
"properties": {
"title-vector": {
"type": "dense_vector",
"dims": 768,
"index": true,
"similarity": "dot_product"
}
}
}
Be sure to replace "search-blogs" with what you named your index. In addition, "title-vector" is the name of the field where the vectors will be stored.
Now that you've created your pipeline, select Crawl in the upper right corner then Crawl all domains on this index.
Finally, it's time to execute a kNN vector search to find the information you need. To do this, you should leverage Dev Tools. Check out the video below to know how.
Next steps
Thanks for taking the time to set up semantic search for your data with Elastic Cloud. As you begin your journey with Elastic, understand some operational, security, and data components you should manage as a user when you deploy across your environment.
Ready to get started? Spin up a free 14-day trial on Elastic Cloud.